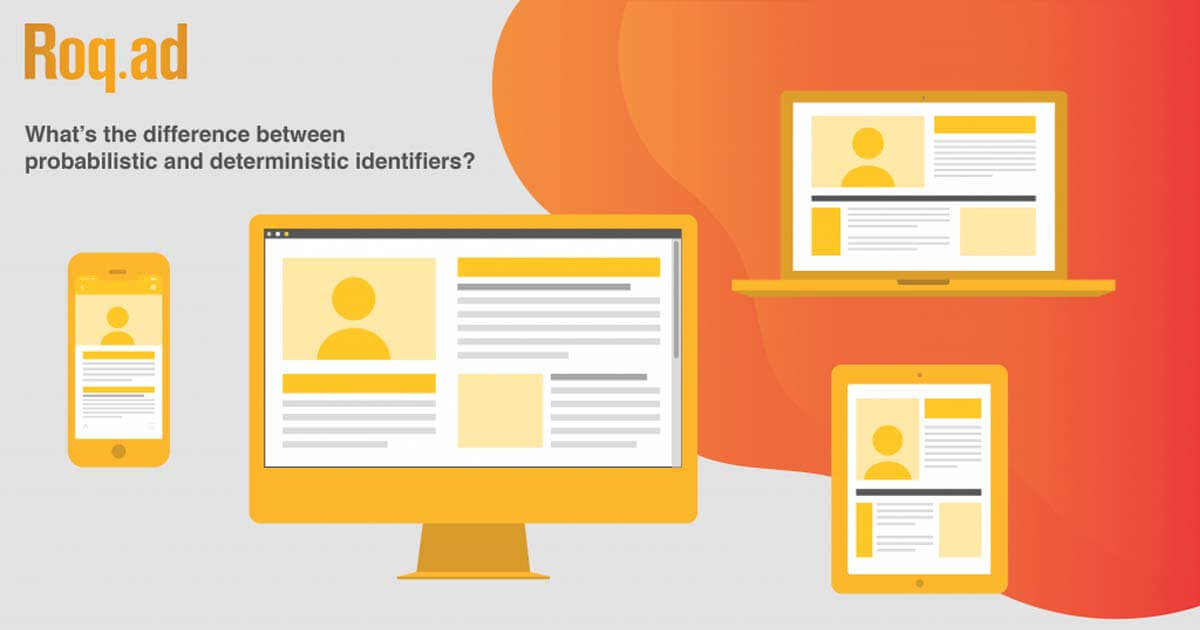
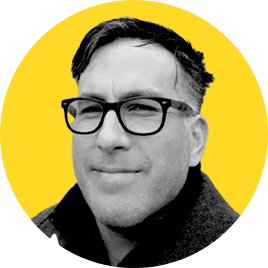
It’s an interesting time for digital and offline marketers all over the world, most of whom are scrambling for alternatives to Google Chrome third party cookies and Apple IDFA.
But the problem of recognizing customers and potential customers pre-dated the so-called cookie and IDFA apocalypse.
Today’s user is connected to the internet using multiple devices – smartphones, laptop, tablets, connected TVs, video game consoles, etc.
This produces highly fragmented data points, which poses a major challenge to marketers to provide contextual advertisements and a personalized experience to consumers.
Enter Probabilistic vs Deterministic Identity matching. These terms and methods have been familiar to digital advertisers, marketers, publishers and ad tech pros for years.
But now there is renewed focus on these methods as many organizations scramble for a foothold in the cookieless future world.
What is Deterministic Matching?
Deterministic matching uses deterministic data to match user profiles.
Deterministic data is information that is known to be true and accurate because it is provided by users directly or is personally identifiable, such as names or email addresses. Deterministic matching scans the data sets and links all user profiles belonging to the same physical person together with a common identifier.
Some of the common deterministic identifiers collected by companies are Name, Address, Email address, Date of birth, and/or Phone number. Many sites today require users to provide a known piece of personally identifiable information in order to access the site’s content and features.
Obviously, deterministic matching has some pros. Because it can be tied to a person, it’s a highly accurate solution and great for targeting and measurement. Additionally, a user’s consent becomes easy to track, which takes care of privacy concerns.
The downside to deterministic matching is this — how often do users actually supply their personal information in order to access a site’s features and content? Estimates for the authenticated web ranges from 10-20% of users supplying personally identifiable information.
If you’re the New York Times, great, then chances are a lot of users will hand over their email address and other personal information to read articles. If you don’t have such strong brand recognition, then suddenly you have a major blind spot in recognizing your users. Scale is a real issue.
There is great value in understanding identity deterministically, and there is likely to be an increase in publishers of using email gates in front of their content so they can use that email address as the key to a deterministic identity service, then get an anonymized ID from that service provider based on the email address to pass as a parameter in the bidstream.
What is Probabilistic Matching?
Probabilistic matching, as the name suggests, is based on probabilities, which is the branch of mathematics concerning numerical descriptions of how likely an event is to occur, or how likely it is that a proposition is true.
The probability of an event is a number between 0 and 1, where, roughly speaking, 0 indicates impossibility of the event and 1 indicates certainty.
In the adtech world, probabilistic data modeling employs a collection of individual pieces of information such as a device’s IP address and location, and then draws a conclusion based on probabilities, which is then used by adtech to create user profiles.
For example, we can look and see two different Android phones and two different Windows laptops use the same wi-fi consistently throughout the week.
We can reasonably conclude that they are members of a household. If we look at which sites each device is used to view, then we can also tell which devices belong to which person.
Android device A and Laptop B both have been used to shop for Gunpla models and used Ramones records; we can probably conclude that they belong to the same person within the household.
Meanwhile, there’s another mobile connected to their wi-fi only on weekends. We can assume that the other mobile belongs to someone who does not belong to the household or is not a full time member.
Unfortunately, probabilistic identity is also widely misunderstood in the industry, according to Digital Content Next.
While it does often rely on IP addresses, this is not the same thing as “device fingerprinting” which is commonly used by fraud detection software (as well as online fraudsters). In fact, probabilistic identity has no more or less privacy burden than multi-page contextual targeting that leverages a first-party cookie.
IAB Europe Transparency and Consent Framework 2.0 stipulates that “with consent, vendors can create an identifier using data collected automatically from a device for specific characteristics, e.g., IP address.”
Probabilistic vs. Deterministic? Nah. Probabilistic and Deterministic!
Both deterministic and probabilistic matching have their unique advantages, and they complement each other by adding value where the other fails.
As more and more consumers start using multiple devices, it is imperative that advertisers start to use probabilistic and deterministic matching to identify users across multiple devices.
The two services can also be used in combination with one another. If eater.com can successfully get an email address for my wife, then they can pass both their first party ID and the Deterministic ID…then the ecosystem can use either, or both tools.
Roqad’s identity resolution graphs helps advertisers to understand the cross-device behaviour of their listeners and optimize their marketing spending across all channels, leading to a user-centric communication.