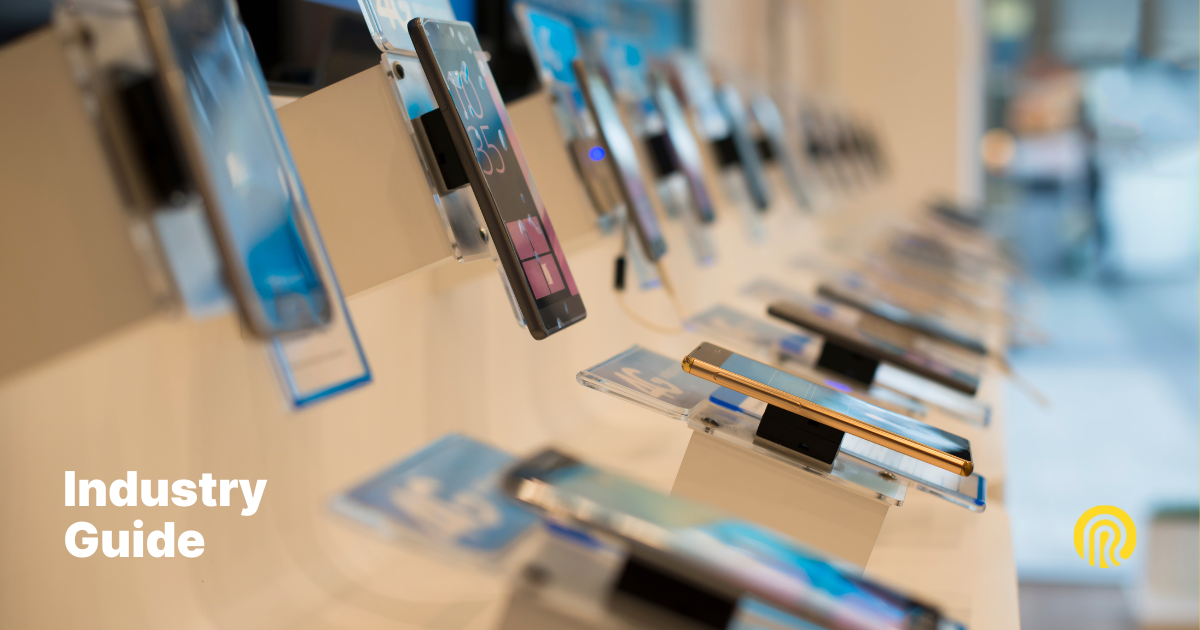
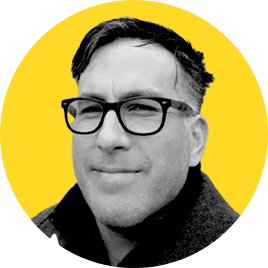
In this piece, we’ll tackle guidance on the use cases for identity graphs in consumer packaged goods.
Consumer packaged goods companies are, in droves, working to generate a much deeper understanding of their customer. They are increasingly bringing their media planning and campaign activation in-house, and allowing for direct relationships with the Adtech community.
As brands make these seismic switches, there are a number of challenges for consumer packaged goods, but chief among them is not owning large swaths of the funnel.
Instead, much of the customer interaction is owned by retailers and eCommerce vendors, with brands left guessing which messaging is actually driving product adoption.
Whether you are selling M&Ms or mobile phones, if you don’t own the last mile of the path-to-purchase (aka: the execution of that purchase) it’s significantly more difficult to do each of the following:
-
Understand who your most likely prospects are (and are not)
-
Identify the most relevant messaging for each prospect type
-
Deliver the correct message to each consumer at the right time
Identity graphs can help with each of these challenges, but most brand marketers have difficulty implementing the tools to address them at scale, especially since many brands see very little direct interaction with their customer.
In this article we will examine some critical use cases of identity data and identity graphs in consumer packaged goods (aka: the CPG space) and how they relate to the goals above.
The objective of this overview is to give CPG marketing teams concrete steps they can work together on to unlock the value of understanding identity at scale without overwhelming their (usually) small data analysis teams.
How does a brand build from the data they have?
In CPG, the data we see is often related to the truly “faithful,” or only the consumers who are most brand-loyal.
It’s pretty rare for someone to go to the M&Ms website even if M&Ms are their favorite candy. Those that do go are the brand’s most ardent fans.
Add to this that one isn’t likely to visit the M&Ms website often, and it puts brands on their heels when they are trying to figure out who their customer really is.
Identity can help.
The faithful are a great group to use as a seed audience, but data about them needs context and stability.
Identity can help with both context and stability by translating those cookies into Mobile Ad IDs (MAIDS) and Hashed Email Addresses (HEMs), each of which can be easily related to deep demographic and psychographic data, interests, etc.
Both are also notably more stable than the ephemeral third-party cookie, so storing data against those stable IDs make it much more valuable in the long run because you will be able to use it for months and maybe years, rather than weeks.
This allows marketers and brand data teams to make decisions over time about their audience, and action those choices through things like lookalike audience generation, and demo/psychographic profiling.
It doesn’t end there though, through a combination of Identity matching and detailed attribution through partners, we can match SKU level conversions in a way that allows us to build more and more data about customers with brand loyalty, that we can push back into the model.
As those initial lookalikes see ads for M&Ms, we can then watch for those in the target group using an attribution partner that has access to SKU level data via loyalty programs and see that the person we are advertising to is buying M&Ms rather than Skittles.
That knowledge feeds on itself over time and becomes an increasingly valuable dataset to drive increased sales by better understanding the customer, even when you don’t have access to a SKU level attribution partner.
How identity builds insight and revenue, step by step (with a snowball effect)
★ Customer Segmentation
- Step one here is to stabilize the audience we have to something we can action: HEMs & MAIDs, for that we take the cookies that come to the M&Ms site and match them to data coming from other sources to the identity graph provider. This gives you a match between what you learned about this user to a MAID & HEM that can be easily uploaded to a DMP or CDP to generate more information on the user.
- Functionally, you build a segment (or segments) that has each of the IDs representative of your faithful audience into your CDP.
- Now that we understand our audience and have built it into segments that we want to target based on the data we ascertained from our own knowledge of the user, we want to add what we can from DMP or CDP partners.
- For this, we restrict our segments to MAIDs and append demographic and psychographic characteristics to further assist in segmentation. After the further segmentation is complete, we re-attach all the non-MAID IDs representative of our target segment via the Identity graph.
★ Lookalike Modeling
- Once we have a good handle on our base segments, we can look at applying machine learning to those segments to build similar audiences that do not include your faithful, but look very similar to them. This allows you to take your known group of say 1 million faithful customers and enhance it to 5 million that look like them.
- From there you will want to pass that larger lookalike audience through your identity graph again to expand the amount of IDs in your lookalike target segment to something like 12 million IDs.
- At each step, you really just bounce each segment you build off of the Identity Service before you take your next action.
★ Detailed Attribution
- The snowball effect starts to take shape when you add in detailed third party attribution to your toolset. These toolsets have access to panels of people who give them detailed loyalty and/or credit/debit card transaction level data that they can match to your lookalike target segment.
- Basically, if you reach an ID in the RealTime bidding, then you send the attribution provider all the IDs known to be owned by that person.
- If any one of those IDs is in their panel, then they will watch for conversion, and tally those that happen. They will also build a projection for the full reach of your campaign based on the ratio of their panel to the population in the area of your campaign.
- Those projections are different if you fire all of the IDs related to a person rather than just the one you actually reached them on, so be sure to work with your attribution provider and make sure that they understand that you are using an Identity Graph.
Conclusions
Adtech companies sometimes make big data projects feel out of reach for smaller CPG analytics & marketing teams.
But they don’t have to be that way.
Yes, an identity graph is a raw data asset, and it takes some massaging to make it really work for you, but the raw power you gain in each use case makes it well worth the effort, and the massaging is often much less significant than it appears at first glance.
More importantly, when you work with Roqad, we’ve done it before. Our team has built and implemented identity graphs for use cases as simple as segment extension for a DSP and as complex as measuring mobile phone churn for a hedge fund, and anything in-between.
We’ve seen it all before. Don’t fear the unknown when it comes to identity graphs in consumer packaged goods. and we can help.
QUESTIONS?
Contact us. We’re here to help.