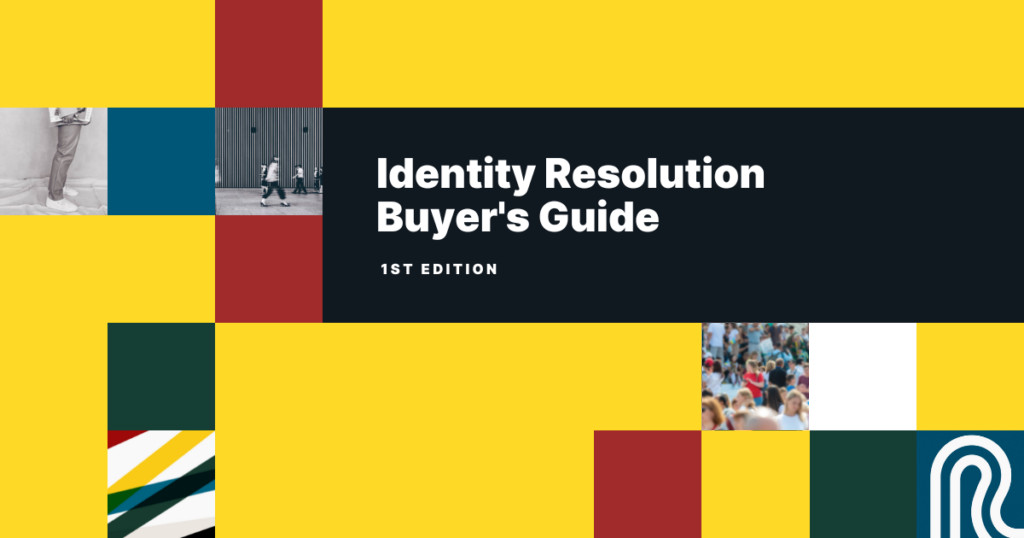
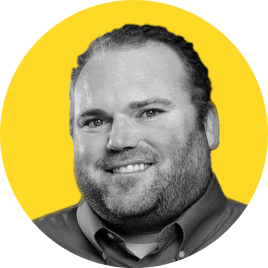
<The 1.3 edition of the Identity Resolution Buyer’s Guide was released on July 26, updated with info about choosing the best process to ingest the identity graph: waterfall or graph of graphs. Download form below>
<The 1.2 edition of the Identity Resolution Buyer’s Guide was updated with info about the VCDPA.>
The most common identity solution on the market is undoubtedly the identity graph, yet the path to purchase can be challenging to navigate.
What should you consider when evaluating and buying an identity resolution solution?
You’re in the right place to find out.
We’re going to get into the weeds in this Identity Resolution Buying Guide to help you make informed decisions that deliver strong ROI for your business.
Get ready to learn about:
- The technical details related to identity resolution solution value
- What you should consider when evaluating graphs and partners
- How to begin your data acquisition journey … and how to bring your company along!
Let’s go!
See below for the TL;DR version, or download the full PDF version here:
Section 1: Identity Graphs Today & in the Future
– – –
What is an Identity Graph?
A probabilistic identity graph maps devices belonging to the same user, creating a cluster (i.e. a fully-connected subgraph).
These clusters correspond to an (anonymized) user and all of the IDs associated with the individual.
Why is this valuable? Visibility to the cluster enables advertisers to target relevant audiences more broadly and effectively which results in better return on marketing investment (ROMI).
An identity graph can also be thought of as a database that houses IDs and data points that correlate with individual users. It is a tool that answers a critical question for businesses that have online operations: Who is this person?
The identity graph incorporates a hierarchy with two major identifiers:
- The Household ID (HHID) – and –
- The Person ID (PID)
And then there are many other minor IDs in each network:
- Hashed email addresses
- First party cookies
- Third party cookies
- Mobile device ID
- Phone numbers
- Account usernames
- Customer IDs
- Publisher IDs
- Intermediary IDs
The PIDs are the “direct reports” of the HHID, as are characteristics that are better served to mapping a household (like address or Connected TV ID).
So a HHID has two, three, or more PIDs and each PID has several ways IDs are tied to it.
Each of those ways to map creates a list of IDs that are connected to the PID and HHID. The data points pile up fast, so a data science and machine learning play a critical role in the mapping process.
A device ID can be identifiers such as a first or third party cookie, IDFA, UID, Hashed Email, CTV ID, App ID, IDFV and an IP address (the list goes on). A 3rd party cookie identifies, for example, a desktop or a mobile web browser, while IDFA and AAID identify mobile devices.
Deterministic vs. Probabilistic
It’s an interesting time for marketers all over the world, most of whom are scrambling for alternatives to Google Chrome third party cookies and Apple IDFA.
But the problem of recognizing customers and potential customers pre-dated the so-called cookie and IDFA apocalypse. Today’s user is connected to the internet using multiple devices – smartphones, laptop, tablets, connected TVs, video game consoles, etc.
This produces highly fragmented data points, which poses a major challenge to marketers to provide contextual advertisements and a personalized experience to consumers.
Enter deterministic and probabilistic identity matching.
These terms and methods have been familiar to digital advertisers, marketers, publishers, and ad tech pros for years. But now there is renewed focus on these methods as many organizations scramble for a foothold in the global cookieless future.
Deterministic data and probabilistic data are opposing terms that can be used to describe customer data:
- Deterministic Data: Sometimes referred to as “first party data”, is information that is known to be true and accurate because it is provided by users directly or is personally identifiable; it is based on unique identifiers that match one user to one dataset.
- For example, the relationship between a MAID and its corresponding Hashed Email is a deterministic pair.
- Probabilistic Data: Information that is based on relational patterns and the likelihood of a certain outcome.
- For example, many identity graph companies will infer a user’s household IP based on the dwell time that user’s MAID dwell time at a specific IP location during the night time.
Both deterministic and probabilistic matching have unique advantages and they complement each other by adding value where the other may fall short.
The two services can also be used in conjunction with one another. If a publisher can successfully get your email address, then that publisher can pass both their first party ID and the deterministic ID…then the ecosystem can use either, or both tools…more options. More potential.
At Roqad, we employ deterministic data as a means to strategize our probabilistic elements.
The difference between a third-party cookie and a first-party cookie:
- First-Party Cookies are directly stored by the website (or domain) you visit. These cookies allow website owners to collect analytics data, remember language settings, and perform other useful functions that provide a good user experience.
- Third-Party Cookies are created by domains that are not the website (or domain) that you are visiting. These are usually used for online-advertising purposes and placed on a website through a script or a tag. A third-party cookie allows companies who are not the owner of the site in question to drop a unique ID token for a browser in that browser’s cookie storage.
The main difference between first and third-party cookies in practice is the setting of the cookie:
- Setting the cookie: a third-party cookie is set or read by a third-party server when the user’s browser makes a call to that third party server. This happens because the code that the browser download from the publisher tells the browser to make that call.
As a standard website is loaded by a browser, it may make many third-party calls for various reasons, like, the photos on the page are stored in a content delivery network (CDN), or a mobile version of the site is hosted by a third party provider, or the they have social networking or chat functionality from a third party. The list goes on and on, but often when you think you are going to one site, you are actually making requests to more than 10.
Once the browser calls that server, the server can look at the browser’s cookie store and figure out if they’ve seen that user before.
What is about to change – with the deprecation of third-party cookies – is that the browser is going to set a limit: the base page (the first URL the browser went to), must match the domain of the call in question to allow access to the browser’s cookie store for reading or writing cookies.
Google’s announcement to do away with the third-party cookie heavily impacts the adtech industry. So, what happens when browsers take away the third-party cookies?
With our dearly departed third-party cookie, advertisers lose the ability to monitor a user’s browsing behavior across sites and in turn, serve targeted ads. As in – any data sharing between companies based on matched cookies to help the targeting process comes to an abrupt end.
This is where Identity Resolution comes into play.
Identity resolution is the collection of the many and scattered data points that are tied to a user, collated into one single view of the user.
A user’s identity data points are scattered and anonymized, and might come from laptop browsers, cell phones, email subscriptions, maybe offline purchase history.
Resolution means the compiling of all of this information into one profile.
Compiling the information allows for vision of people, as opposed to just devices. It allows for personalization of offers and measurement of performance in campaigns. The means for compiling is the identity graph.
Identity Resolution with Roqad
A lot of adtech vendors that operate in the EU say that they respect privacy, but we’ve gone a step further and baked it into the Roqad public and private identity graphs.
Graphs are generated for each customer that include only IDs that have consent for our Vendor ID & the clients’.
It’s called “2-way TCF consent.”
TCF stands for Transparency Consent Framework, and Roqad was an early adopter of it. We’re actually #4 on the TCF vendor list (and there are more than 800 IDs as of today).
***
Section 2: Use Cases & Geographic Specificities
– – –
What types of challenges do identity graphs address in your business.
To buy an ID graph, it is first important to outline your use case.
Within adtech, many different types of businesses find value in an ID Graph. Businesses like DSPs, DMPs, CDPs, Publishers, Agencies, brands and more.
Here are some of the use cases we commonly address in the consultation process.
Identity Graph Use Cases:
- SEGMENT EXTENSION: Either to reach customer audiences for retargeting or to apply to a client’s broader segmentation strategy, segment extension adds by improving the accessibility of your audience. Generally, the pools are built such that they can be reached across all devices with a specific message. Reaching more members of the audience in more “places” results in better campaign performance. Roqad stitches IDs together so that you can apply what you know about an ID to any other matching ID in the graph. Think of it as a way to apply what you know to your logged in audiences across a huge number of the unknown IDs that you see, because they are actually the same person.
- SEGMENT TRANSLATION: This is really a subset of extension, and is technically done by extending a segment to all known IDs related to users in those segments, then shrinking it down to the ID type(s) you specifically want to target.
- ATTRIBUTION: This is the flip side of the targeting coin. First you must find the target with segmentation strategies. Then, you need to know where to place credit for target audience members who take the desired action. Let’s say you’ve targeted someone with a video ad for a new vehicle, identity graphs solve the attribution challenges associated with the two main KPIs for such an ad placement:
-
-
- 1) Conversion to building a new truck online (cookie based desktop conversion)
- 2) Visit to a dealership (MAID based mobile conversion)
-
- ANALYTICS & MARKET RESEARCH ENGAGEMENT: By understanding what IDs you’re able to action and serve an impression against, you can understand the behavior of users and the best way to target them in the future.
We often see more specific use cases for various subsets of our customers:
- Ad networks & SSPs who implement an ID graph typically have some level of data attached to their existing business and look to resolve identity to optimize impressions served and command higher CPMs. Think of an identity graph as a giant spreadsheet, where each row represents a single individual. The first column of each row is the unique home-base ID. All additional columns are “other” IDs that have been collected by the graph provider and resolved back to that person. In the case of Roqad, we do this based on our machine learning algorithms. On average, we collect 4-5 IDs per person, but we estimate that number will rise to 25+ after third-party cookies deprecate. Why does this deliver business value? Let’s say you’re offering up an impression on site A at a $1.00 CPM. You can command a higher price if you have valuable data connected to that impression. Perhaps your data that shows the person behind the impression is Full-Time employed, Owns their Own Residence, Leases a Luxury Vehicle, and has been recently Shopping for Diamond Engagement Rings online. Tomorrow, however, the person behind that impression goes to site B for the first time ever. Without an ID strategy to bring the two impressions together, you’ll never know that they are attributable to the same person. Our probabilistic graph will allow you to tie the site A publisher User ID to the site B publisher User ID in an automated way. The net effect of this will be that the SSP earns a higher CPM on both impressions.
- Agencies often look to ID graphs for media planning and optimization.
- Unique applications: Clever clients often bring nuanced use cases to us and we work closely with them to help deliver unique solutions. accommodate our clients with nuanced use cases.
Shifting Market Dynamics Value Proposition & Positioning:
Three significant changes are taking place in digital marketing:
- Increased consumer interest in privacy, transparency and control of personal data
- Increased regulation governing control and use of personal data by technology companies
- Strengthening of the so-called “walled gardens” of Google, Amazon, Facebook and Apple. Important actions by Google and Apple include:
- Apple launches ATT which encourages users to opt-out of IDFA tracking
- Apple launches Private Relay – currently subscription only
- Google announces delay to third party cookie deprecation from 2022 to 2023
As a result of these changes, Adtech companies are trying to support independent solutions to enable privacy-safe tracking and targeting. The launch of various new Universal Identifiers from different vendors is a response to the changing landscape of privacy regulations and the actions of the walled gardens (GAFA). The introduction of Universal Identifiers has created a more disjointed understanding of the user journey.
In response to these changes, Roqad’s Graph is able to provide you a map of an individual user in which we give you all of the digital IDs we have resolved back to that person. Our coverage in North America and Europe provides you access to a scalable solution to these industry changes.
Factors to Consider as a function of Geography
One of the most important geographic considerations for your identity strategy is consumer data privacy.
Governing laws such as CCPA in the US and GDPR in the EU dictate the fundamental ways in which advertising technologists can work with data.
Roqad was born out of desire to support the programmatic advertising community with respect for GDPR. There was a critical opening in the EU market for a GDPR compliant, probabilistic graph.
With regulations rolling across the US state-by-state and with refinements being made to the way data can be used around the world, it’s important to keep geographic considerations front and center in your graph buying journey.
- Necessary server spaces in EU for accessing EU data
Roqad Solves Identity Challenges Today, Tomorrow and In Any Future State
- ID agnostic – Roqad’s unique approach is to stitch together all existing and new cross-device identifiers and not to become another universal ID.
- Future proof – Roqad is inherently future-proof and will continue to thrive as an identity data vendor or choice as the landscape changes. Our data experts know what happens when CNAME access goes away and our premiere solution for cross device attribution and segment extension will continue to support customers needs without interruption.
- EU:
- Privacy-by-design –Roqad has taken a unique privacy-by-design philosophy to build systems and processes that have consumer privacy and control at the center.
- IAB TCF consented data only – Roqad only uses data that has a TCF consent string attached. This applies to all providers of data including client data-sets.
- IAB TCF 2.0 info: https://iabeurope.eu/tcf-2-0/
- US:
- We are conscious of the CCPA and positioned as an early adopter as new states go live with consumer data privacy regulations.
- EU:
***
Section 3: Your Discovery Phase
—
Discovery Call:
When you begin speaking with identity graph providers to determine alignment with your needs and the potential for partnership, you will likely kick things off with a discovery period. This will be comprised of calls and information trading between you, your buying committee and the vendor team.
During our initial discovery call, we typically set an agenda and engage with prospective customers to understand the critical business objectives tied to identity value, technical parameters of importance and the priority level such an identity initiative has compared to other projects that will compete for the time of the prospective client’s team.
From there we can clarify a use case and propose how various solutions will best address the need and provide optimal value for the stakeholders involved.
Ideally, you will have solicited questions and concerns from your buying committee in advance of initial discussion so you can ask a potential vendor, in this case Roqad, all of the critical questions for your own discovery process.
We recommend starting with topics to include, but not be limited to:
- Data / Graph:
- Scale
- Geographies
- Privacy approach
- Data inputs and outputs
- Does the graph rely on a critical owned ID with the graph provider or is the graph provider ID agnostic now and in the future?
- Industry Expertise:
- Does the vendor clearly and easily understand your use case?
- Do the vendor’s SMEs have a clear understanding of industry terminology?
- Review the credentials of the most relevant executive team – ie: Chief Product Officer, CTO, etc.
- Ask how they stay up to date and compliant with consumer data privacy regulations around the world.
- Ask yourself and your own company:
- Do you know what you will do with the data provided when you have it? Do you have the systems, processes and people to take the necessary action to gain value?
Depending on the predicted value and fit, a MAP (or mutual action plan) will be reviewed in order to align all involved parties to the needs and timelines associated with your buying journey.
We’ll talk about the week by week action items required to get you live fast.
Getting your Buying Committee on Board
Ideally, your company is fired up and ready to put the necessary time and attention into an identity project.
However, this isn’t always the case.
If you’re a key stakeholder, but not the ultimate decision-maker, take these steps to turn your passion project into a company-wide (ok, maybe not company-wide) priority.
- Steps to gaining executive support for and identity product solution:
- It’s important to recognize that Identity is an innately heavy topic that will require the permission and support for implementation from a CPO. Similar titles that often dictate a say include Chief Data Officers, Chief Strategy Officers, CROs & CEOs.
- Take the necessary steps to begin the education process internally, or bring in a partner like Roqad, to work with you on a buying committee conversion strategy. We can help to educate them on the initiative and value if you’re less than clear on how to make this happen on your own.
- Committing to an evaluation takes engineering work for both companies involved. Be sure to include the people who will be responsible for working on the graph project from the beginning so your project is not derailed due to a lack of clarity on behalf of your internal team about the technicalities and timelines involved.
- Take it from us, put everything in writing. Do you have a slack channel or a project management board? Invite all of your internal stakeholders to follow along. Tell them what you’re going to do, tell them as it’s happening, and then at the end tell them what you did (it’s like a good thesis paper).
- Incase it wasn’t clear yet – OVERCOMMUNICATE at every turn
- Make it clear to the people involved what is expected of them in terms of timelines to evaluate data and to commit to licensing agreements along the final steps of the buying journey.
- People get promoted all the time for running impressive projects…why not you?
- Once you’ve gone live, continue to advocate the value of the data (how you’re addressing your use cases) for continuous support.
- It’s important to recognize that Identity is an innately heavy topic that will require the permission and support for implementation from a CPO. Similar titles that often dictate a say include Chief Data Officers, Chief Strategy Officers, CROs & CEOs.
***
Section 4: Important Legal Considerations
– – –
CONSIDERATIONS IN THE UNITED STATES
CCPA
The CCPA is the California Consumer Privacy Act, which secures and legislates privacy rights for California consumers.
There are four fundamental rights that every California consumer can exercise if needed:
- The right to know about the personal information businesses in California collect about them and how it’s used.
- The right to delete personal information collected from them (with some exceptions);
- The right to opt-out of the sale of their personal information; and
- The right to non-discrimination for exercising their CCPA rights.
Moreover, businesses must provide transparent information about these issues and offer them the possibility to opt out.
But there’s more.
Any California resident can ask a given company to disclose personal information about them, inform what they do with it, and even delete it if they feel necessary.
Each consumer also has the right to know what kinds of personal data a specific company gathers and processes.
What Constitutes Personal Information in CCPA
In general, it’s everything that can identify you (or your household) as a specific person.
Personal data is at hand even when a given piece of information can be related or linked to a specific customer. So in practice, personal data, as CCPA understands it, involves the following:
- Full name
- SSN (social security number)
- Email address
- Fingerprints
- Geo-location data
It’s also personal information if it could be used to create a detailed profile about your preferences and characteristics. On the other hand, everything that’s publicly available from federal or local records does not constitute personal information under CCPA.
Who Needs to Obey CCPA
CCPA is very specific about what kinds of companies and organizations need to comply. It applies to all the businesses operating in California that:
- Have a gross annual revenue of over 25 million USD
- Buy, process, or sell any personal data of at least 50,000 California residents, households, or devices
- Derive 50% or more of their annual revenue from selling California residents’ personal data
If your company meets at least one of these conditions, you must apply proper operational principles under CCPA.
Unlike many other data privacy and security statutes, the CCPA also carves out from most or all of its provisions:
- Non-profits that do not operate for “profit or financial benefit.”
- Financial institutions that are regulated under the Gramm-Leach-Bliley Act.
- Consumer reporting agencies that are regulated under the Fair Credit Reporting Act.
- Health care providers that are regulated by the Health Insurance Portability and Accountability Act
Data Breaches in CCPA
Of course, a data breach can happen at any time. However, consumers cannot use any data breach to sue a company.
There is a whole list of conditions that need to be met. Consumers can file a lawsuit only if their full name was stolen in combination with their (at least one):
- Unique identification number (e.g., SSN number, driver’s license number, passport number)
- Financial account number/credit card data
- Medical/health/insurance information
- Biometric data
Furthermore, all of that information must have been stolen in a non-encrypted and non-redacted form. All in all, it’s rather unlikely for such a massive and uncontrolled data breach to happen.
New regulations are rolling out state-by-state in 2023 and it’s important to work with an identity parter that understands and respects these privacy
CONSIDERATIONS IN EUROPE
GDPR IN THE EU
The Difference Between CCPA and GDPR
The General Data Protection Regulation (GDPR) was introduced back in 2016, and it is a legal framework that sets guidelines and regulations concerning processing personal data coming from individuals living and working in the European Union.
When compared to CCPA (as discussed above), GDPR is a much more complex and broader privacy protection law.
In short, the main rule in GDPR is called “privacy by default,” and it means that for a company to process personal data, it needs to have prior consent from a specific person.
Under GDPR, Europeans have a range of rights, including the right to access, erase, and modify their personal information.
Furthermore, they almost always have the right to withdraw their consent to process personal data. CCPA is, without a doubt, a more specific law and less .
GDPR Principles
Let’s go further.
GDPR provides six legal reasons to process personal data…
Whereas CCPA doesn’t give any. This means that businesses in California can process personal data however way they want and for whatever they want. All they have to do is provide the opt-out procedure.
GDPR protects any consumer who is in the European Union at the time of collection or processing (they don’t have to be residents of the EU).
On the other hand, CCPA only protects California residents.
Another difference – CCPA deals with personal information that identifies, relates to, describes, or links with a consumer or household.
GDPR deals with any personal data of an individual but does not include households. Only anonymized data is exempt.
And the last thing that is worth mentioning is penalties.
Both legal acts have some penalties for practices that go against their guidelines.
However, the European regulation is much more strict here. The penalty can go up to 4% of the company’s global annual turnover.
When it comes to CCPA, there is a maximum penalty of just 2,500 USD per violation (or 7,500 USD in case of international breaches).
Benefits of TCF v2.0 (relevant to the EU audience)
Transparency and Consent Framework (TCF), was created to help all parties to display and manage digital advertising and develop targeted content complying with the the European Union’s (EU) General Data Protection Regulation (GDPR) and Privacy Directive (ePD) when processing personal data and or accessing and storing information about users’ devices.
GDPR was assembled to protect users, but it doesn’t easily lend itself to implementation. That makes the TCF accomplishment all the more commendable.
Roqad was an early adopter of TCF. In fact, our Vendor ID number is 4 — proof that we saw the value of this project early on.
We spotted that the mission of the Interactive Advertising Bureau aligns with Roqad’s values, especially “Privacy by Design.”
The first version of the TCF was launched in April 2018. It provides a means of transmitting signals of consent from users to vendors working with publishers using a Consent Management Platform (CMP).
Specifically, TCF v2.0 supports the following new features:
- Transparency – through revised definitions and descriptions of data processing purposes that combine greater granularity (now increased from 5 to 10 purposes with the addition of 2 special purposes, and 2 features and 2 special features) that will enable users to make more informed choices regarding the processing of their personal data.
- Choice – the introduction of signals that allow CMPs to offer users a streamlined means for users exercising the “right to object” to processing on the basis of a “legitimate interest.”
- Accountability – with a more complete accommodation of the “legitimate interests” legal basis for data processing that allows vendors to receive a signal about whether their legitimate interests have been disclosed.
- Control – with new, granular controls for publishers about the data processing purposes permitted by them on a per vendor basis.
- Compliance – through greater support for the users of the framework in their application of the policies, terms and conditions and technical specifications with increased investment by IAB Europe in the resource to support compliance.
Controller, Processor, Subprocessor Dynamics
What is the relationship between your data, as a client, and Roqad’s data with respect to GDPR (as well as guidance provided by the United Kingdom’s Information Commission Office)?
We made the chart below to help clarify the matter.
Some of the keys to locating your organization on the chart:
- Are you using Roqad’s Private or Public Graph?
- Are you passing Transparency & Consent Framework Strings to Roqad?
The UK ICO has told us you cannot be both a controller and a processor of:
- The same dataset
- For the same purpose
- At the same time
This guidance means that if Roqad ingests customer data and combines that customer data with data from other sources, since it is a controller on the data from other sources, it is a controller on everything.
That controller status triggers an Article 5 requirement to verify consent on the data coming from the customer.
As an alternative, we can keep the datasets separate.
In this scenario, Roqad matches IDs with the customer per a customer pixel fire (customer is either a controller or a processor on behalf of an end client who is a controller; Roqad is a processor or a sub-processor).
Option A: a pre-post-cookie deprecation example:
- Dataset #1: cookie match table that matches Roqad cookies to Partner cookies (Partner is a processor and Roqad is a sub-processor)
- Dataset #2: public graph with everything relevant in the Roqad cookie space (with most recent TC Strings) (Roqad and Partner are both Controllers)
This allows us to provide the Roqad customer with the following:
- Dataset #1: Partner ID <> Roqad ID
- Dataset #2: Roqad ID <> Other Extended IDs
Option B: a post-cookie deprecation example:
- Dataset #1: public graph with ONLY Partner cookies as an output. (Roqad and Partner are both Processors)
- Dataset #2: public graph with everything relevant EXCEPT Partner cookies (with TC Strings) (Roqad and Partner are both Controllers)
This allows us to give a Roqad partner the following:
- Dataset #1: Partner ID <> Roqad person ID
- Dataset #2: Roqad person ID <> Other Extended IDs
Option B may not see the conversion until a future graph build, because we may not have mapped the partner cookie until the conversion happens.
But we should be able to generate a significant amount of conversion lift for the Partner over click through rates in a post third-party cookie world.
Other Governing principles: DAA, NAI
- Networking Advertising Initiative
- Digital Advertising Alliance
Privacy
The Right to Opt-Out
We understand the request to stop selling your personal information by this short term.
There are some exceptions, but once a company receives such a request from you, they need to stop selling it immediately (of course, unless you authorize them to do so again in the future).
With regard to this law, businesses operating in California need to provide a transparent “Do not sell my personal information” link directly on their website.
It has to contain a form enabling any person to submit (unconditionally) an opt-out request.
Legal Business Terms
Order forms + DPAs: Since Roqad is rooted deeply in respect for rapidly changing privacy laws – we have built out templated contracts to quickly produce this before we undergo any POC or data transfer.
Typically, this entails at least one Order form (for each graph or product) and an additional DPA that will cover any data transfers mechanisms for the EU abiding by GDPR.
Section 5: Evaluation and Metrics
– – –
Data onboarding is taking offline or CRM data that’s tied to home addresses or hashed emails and connecting that data with online identifiers such as mobile ad IDs (MAID’s) and cookies.
Data onboarding strengthens and activates customer databases.
And for some companies, data onboarding is the one thing that stands between it and catastrophic loss of data viability and investment, depending on the format of their hashed email addresses.
If using an older hash format, definitely have a look at one of the data onboarding offerings out there.
What Is an Email Hash?
So what is an email hash or “HEM”? LiveIntent offers a helpful description: an email hash is a code created by running an email address through a hashing algorithm. Whenever you log into a website using your email address, you are recognized throughout your session.
Hashes are a standard across the web. This code (i.e., hash) cannot be reversed, making it a completely anonymous customer identifier.
Here are some types of hashing algorithms:
- MD5 (message-digest algorithm): The output will always be 32 characters. In recent years, MD5 has lost popularity to the SHA family of hashing functions.
- SHA-1 (secure hash algorithm 1): Designed by the United States National Security Agency as a Federal Information Processing Standard. The output is a hexadecimal number 40 characters long.
- SHA-2 (secure hash algorithm 2): The successor to SHA-1, the SHA-2 family consists of multiple hash functions with hash values that vary in size, the most common being SHA-256, which produces an output of 64 characters.
The benefits of email hashing include the fact that the email address is a relatively stable ID that represents a known customer. It is often unique to an individual and has a degree of persistence across devices, apps, and browsers.
Consumer email addresses are transformed into anonymized identifiers that cannot reveal any personally identifiable information, making the email hash a valuable people-based identifier.
Let’s spin the clock back 25 years.
You run a company in the mid-nineties. How do you gather information about your customers?
Most likely, you use simple offline databases, perhaps based on MS Excel. And when it comes to identifying your customers, you have to operate with offline identifiers, such as:
- Name
- Email address
- Physical address
- Phone number
These identifiers are, of course, still in play today.
And in the cookieless world, their importance has grown significantly. With data onboarding, you can take that offline data online and make the most of it in the digital environment.
In order to make this “merging” possible, there has to be a match between the specific user’s profile and their online activity. Data onboarding provides such a match and enables you to see your customers online.
Let’s go back to our 1990’s company example.
Imagine you wanted to send a new catalog to your customer base in the 1990’s. It was pretty straightforward back in the day. You just had to open your Excel database and copy and print all of the physical addresses.
In today’s digital world, this matter is a bit more complex. Until recently, third-party cookies and Apple’s IDFA have played the role of the primary online identifier.
And shortly, companies all over the world will have to redesign their addressability solutions since IDFAs are disappearing and third-party cookies won’t be available anymore. Where does it leave us?
There are new identifiers that can be used for a similar purpose:
- Unified ID 2.0
- Zeotap ID+
- Lotame Panorama
- Various publisher ID’s (mobile apps, connected TV IDs, websites, etc.)
One of the main use cases of data onboarding is connecting CRM or offline data with one of the above new identifiers (and third-party cookies, at least until some time in 2022).
At the conclusion of this process, a company’s CRM data has been activated.
How Does Data Onboarding Work?
Generally speaking, the data onboarding process is based on three steps:
- Uploading data (your company’s first-party data is anonymized and uploaded to your onboarding’s partner system)
- Matching data (the data you uploaded is matched with specific online identifiers)
- Activating data (the last stage is to create addressable audience segments that you can target with your ads and messages)
Of course, you will need a data onboarding partner that will help you execute the whole process.
In their Google Attribution 360 white paper, Google explains what this process looks like from their perspective.
How Can You Select the Best Data Onboarding Partner?
For starters, ask your future partner how long it will take to activate data. In today’s dynamic world, speed is of paramount importance. Users use different channels and devices to interact with your brand and offer.
If you can’t keep up the pace, you will surely lose lots of potential possibilities to interact with users and engage them in your brand’s message.
Secondly, pay attention to data accuracy. Verify your partner’s addressability capabilities (based on authenticated data). Partner with a company that deterministically and probabilistically matches customer data.
And finally, take care of your datasets. Your data onboarding partner should ensure full transparency when it comes to how your data is collected and used. Moreover, you ought to maintain full control over it.
In their whitepaper, Google advises to take a closer look at these additional elements:
- Is their methodology transparent? Do they use proven technology and solutions?
- Can they provide you with measurable results and a successful track record?
- Do they have the necessary privacy and cybersecurity measures in place? Do they use consented data?
Getting answers to all of these questions is critical and will help you make an informed decision.
Evaluating our Graph
There are two ways to test cross-device matching solutions:
- Supervised
- Unsupervised
The former can always be used even without any test data, and its main purpose is to check the overall structure of the graph.
The latter requires a separate set of so-called deterministic data from which the true connections between devices are to be extracted.
Unsupervised Metrics
Typically, when using a cross-device matching solution, you would query an identity graph with a set of device IDs (e.g., provided by a client or partner).
As an output you would obtain a subgraph consisting of all clusters, which contain at least one device ID from the query.
This process is visualized in Fig. 2. The initial identity graph query contains a total of 6 devices:
A, B, C, D, E, and F. By applying the cross-device identity graph logic, you would get a subgraph with 3 cliques, or as Roqad refers to them “Clusters” (clique in the graph theory sense, not mouse “clicks”… just checking if you’re paying attention):
- Note that device F is missing in the response, which means that this device hasn’t been found within the graph
- Device E has no connection to any other devices
- Devices C and D belong to one user and form a clique
- Devices A and B belong to the same user who also owns device M
Based on this output, you are able to compute several metrics. Overlap, which describes how many devices from the query are found in the graph, can be expressed by a fraction of overlapped devices to all devices included in the query. For this example, the overlap is ⅚ (i.e., five device IDs are also contained in the identity graph).
Once satisfied with the overlap, you can then check the number of device IDs from the query that are connected to each other (i.e., shared by the same user). This process is known as deduplication. It is expressed as a fraction of deduplicated devices to all device IDs included in the query. From the same example, the deduplication rate would be 4/6 since the following connections (A, B), (C, D) were identified and contain in total four devices from the query.
You can also measure whether other device IDs already exist in the graph, which are then connected to the devices from a query. These devices already found in the graph then enrich the input set.
This metric is known as enrichment and can be expressed as a fraction of enriched device IDs to all device IDs included in the query.
In our example, it would be 2/6 since only two devices from the query are connected to a new device (i.e., A and B are connected to M).
You are also able to derive other insights from the identity graph, such as statistics focusing on the number of users and/or devices found in the graph, as well as the number of connections between different types of devices (e.g., mobile-desktop).
A histogram of the number of users with a given number of devices can also be easily obtained. These additional statistics further depict the structure of the graph. Keeping with the same example, the relevant histogram is visualized in the left plot of Fig. 3.
For real-life cases of utilizing real data within an identity graph, it is not unusual to see a large number of singletons (i.e., users with only one device). Additionally, some cliques can also be extremely large. In a well-designed identity graph, the number of devices per user should range from 2 to 15, and the number of users with 2 or 3 devices should be the largest. The right plot of Fig. 3 shows an exemplary histogram obtained on real data.
Ideally, each clique in the graph should contain different types of devices, as often found in the real world. There is also a common assumption that each individual user has access to one or two mobile devices as well as a desktop computer.
Therefore, the number of mobile-mobile, mobile-desktop, or desktop-desktop connections in a graph can be an additionally intuitive metric used to depict the structure of an identity graph.
In specific cases where a clique contains multiple desktop IDs only, you can suspect that the graph has deduplicated different IDs coming from one desktop device.
Table 1 shows exemplary numbers of different types of connections in the identity graph.
Supervised Metrics
In the previous subsection, you may have noticed that predictive performance of an identity graph was not mentioned. To be able to measure this form of performance, you would need to have a separate test set of deterministic data from which you could then extract true connections between devices.
Since obtaining ground truth data is usually difficult, your cross-device identity graph provider should be able to run the test using its data. In this specific case, you need to ensure that the users and their devices used for the test have not been touched while training the machine learning algorithms.
Otherwise, the results obtained will reflect an overly optimistic output than what you would experience in reality; therefore, it is essential that the same data should not be used for both training and testing purposes.
At Roqad, we ensure that these experiments are performed with 100% transparency.
The predictive performance of identity graphs can be measured in terms of precision and recall. Precision is a fraction of predicted matches between devices that are the actual/true ones, whereas recall is the fraction of total actual matches that are correctly identified. Precision and recall can be explained visually in Fig. 4, where true connections are the actual ones, and the positive connections are those that are predicted as positive by an identity graph.
The intersection of true and positive connections are called true positives (TP). False negatives (FN) are the true connections that are not predicted as positive, and false positives (FP) are positive connections that are not true connections. Mathematically, precision and recall are defined as
Achieving the ideal balance between precision and recall is one of the main goals of device matching. This balance can be obtained by a harmonic mean of these two metrics and the result is often referred to as an F1 score:
To compute the performance metrics mentioned, you need to have a list of ground truth connections (GTC) obtained from deterministic data and a list of connections from an identity graph (IGC). In both lists you will use only those devices that are in the intersection of deterministic data and the IG. For instance, let us use the same IG as before, containing devices A, B, M, C, D, E, H, G, I, J, K, and L.
Let us also assume that the following devices are included in the deterministic data: A, B, C, D, E, F, G, X, and Y. Fig. 5 recalls the structure of the identity graph and presents the graph based on connections from deterministic data. The intersection of both lists of devices is: A, B, C, D, E, and G.
The GTC list contains four connections extracted from the deterministic data and the IGC list has two connections. These connections are listed in Table 2. To avoid duplicates in the lists (e.g., “A, B” and “B, A”) we assume a lexicographic order of device IDs in each connection.
You need to remember, however, that you should not expect the ground truth connections to be a perfect reflection of the real world, since this information is also obtained from logs.
Therefore, we might not see all connections between devices and observe many singleton users (in the example, we have two such users).
By using the lists above, the computation of precision, recall, and the F1 score is straightforward. Thereby you can compute TP, FP, and FN by using set operations (we use ∩ to denote set intersection and \ for set difference):
TP = GTC ∩ IGC
FP = IGC \ GTC
FN = GTC \ IGC
Table 3 provides the values of performance measures for our example.
Please note, however, that one needs to be very careful in comparing the values of these measures obtained on different test sets.
This is due to the fact that precision decreases as the number of users included in a test set increases. This is because the number of possible connections increases quadratically with the number of users. The same concerns the number of FP.
In turn, TP and FN grow linearly with the number of users, therefore recall is much more stable as it is computed solely on TP and FN. It should be constant for different numbers of users in the test set.
Fig. 6 shows the empirical results of precision and recall obtained on a test set with a varying number of users.
Figure 6: Precision and recall for a test set with a different number of users. The x axis shows the percentage of users used for computing precision and recall.
Based on the above discussion, a natural question arises: what are the right metrics for testing predictive performance of an identity graph?
Precision and recall seem to have become a standard, but the shortcomings of precision are not often discussed. Of course machine learning offers plenty of performance measures that are regularly used for testing predictive models. Unfortunately, many of them are not useful in testing cross-device identity graphs
For example, accuracy and AUC (i.e., area under the ROC curve) strongly depend on true negatives (TN), or “no connections’ between devices that are also predicted as “no connections’ ‘ (i.e., as negatives). Since there are much more unconnected pairs of devices than the connected ones (the number of ‘no connections’ grows quadratically with the number of devices), and the true connections are actually the ones we care about, it would be very confusing and misleading to use these types of metrics.
For example, accuracy is defined as a fraction of TP and TN to all possible connections. Since TN will dominate all other quantities, accuracy will always be very close to 1.
Let us finalize this section by underlining that there is still room for new metrics and methodologies for testing performance of identity graphs. Roqad works intensively on delivering the right methodology for this purpose.
You are now equipped with the basic measurement tools to help you evaluate a cross-device identity graph’s performance using unsupervised and supervised metrics.
It is important to remember that testing cross-device identity graphs is in general quite tricky since the number of possible connections between devices grows quadratically with the number of devices in the test set. Therefore, it is not straightforward to compare results obtained on different, distinct data sets.
Download the full Buyer’s Guide here: