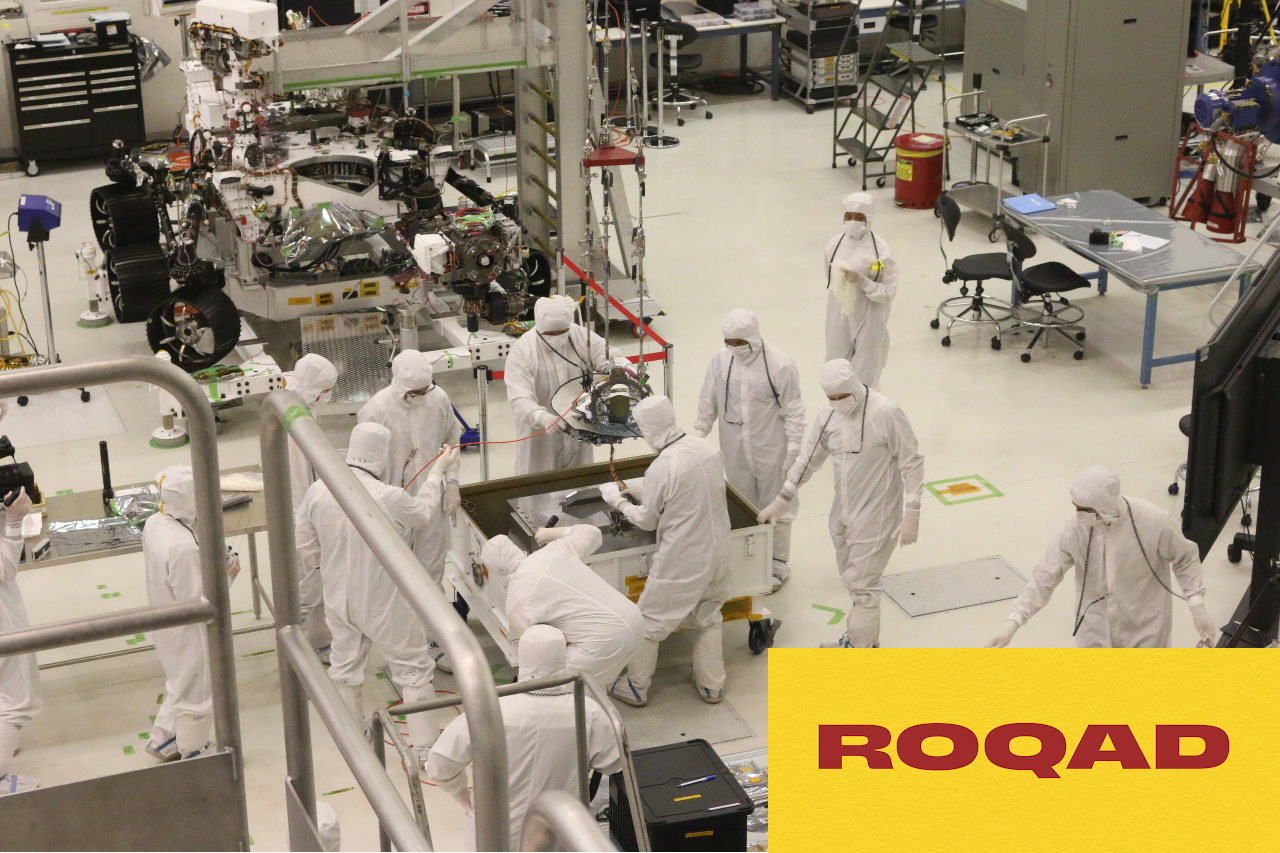
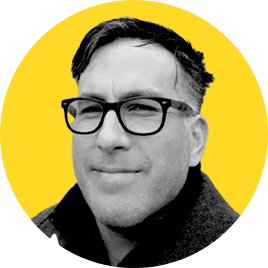
Data clean rooms: not a new concept. Over-hyped? Maybe. Helpful? Definitely.
A virtual event hosted by Roqad: How Consumer Brands Use Data Clean Rooms to Scale Digital Marketing –> January 25, 10AM Eastern Time – UTC−05:00. Register today: https://zoom.us/webinar/89139081275
Data clean rooms have received a lot of attention recently, but they’re not a brand new concept. Google launched their data clean room “Ads Data Hub” in 2017. They were followed quickly by both Facebook/Meta and Amazon. You might have heard of those companies, famous for their beautiful, walled gardens.
Our customers have started asking for data clean room services, so we decided to jump in. Data clean rooms don’t solve every single identity problem in the universe, but they do a very good job with certain use cases. We’ll take a look at the challenges that marketers face and define data clean rooms and how they can help. Put another way,
Question: Who can benefit from data clean rooms?
Answer: Companies that are focused on building a first-party data asset, but understand the importance of leveraging other data to gain a more comprehensive picture of their customer.
Many businesses in the past may have been hesitant to share their rich data assets due to privacy concerns or data leakage (understandable). But the space and offerings have improved, and data clean rooms now offer a vehicle for those companies to do so in a secure and privacy-safe way that benefits them, their partners, and their customers/users.
We’re challenged by challenges: data deprecation, an avalanche of IDs, new privacy regs, etc. etc.
You’re probably familiar with this list, but just in case, here are some of the main challenges facing companies that operate all or part of their business online:
* Consumer behavior fragmented across digital and physical channels and environments (you should check out our case studies for examples)
* Privacy regulations firmly established in the EU and North America, and many other countries are creating their own as well (it’s a good thing, but it does create challenges, or, put another way, a need for new, innovative initiatives!)
* Data deprecation limits access to valuable partner data reducing the addressability, scale, and relevancy of your data-driven strategies (this one hurts, as many of you are well aware)
* Customer-centric companies have a need to collect more and more sensitive data (after all, users and customers want both personalization and privacy protection, creating interesting challenges)
* A continual avalanche of IDs, attributes, and behaviors
The above, left unaddressed, turns into loss of performance – lower match rates, reduced accuracy, poor customer experiences, increased costs.
What can be done?
Data clean rooms can help. Great. So what is a data clean room?
Forrester defines a data clean room as “a secure platform where brands can access advertising data and use it for targeting, measurement, and analysis. The advertising performance data provided in a clean room is aggregated and has controls to ensure privacy.”
Rather than passing sensitive data back and forth directly, organizations share their data securely with a clean room—which then reports back high-level findings about the data to both sides.
Here’s another data clean room definition:
“Data clean rooms are privacy-based technologies that allow companies to merge and match two or more first-party data sets in order to create a new audience or analytics segment informed by both data sets – but without ever allowing either side’s personally identifiable information to be exposed to the other.”
The last sentence is crucial to understanding the value of data clean rooms – without ever allowing either side’s personally identifiable information to be exposed to the other.
It’s a privacy safe way to evaluate advertising initiatives and gain marketing insights.
Here is a definition from Infosum:
“Data clean rooms provide a complete persistent view of the customer without requiring data to be moved into a centralized location eliminating the risk of exposure and leakage.”
And Justin Langseth from Snowflake made a list of features that a data clean room must, by definition, support.
Data clean rooms must control the following:
- What data comes in
- How the data in the clean room can be joined to other data in the clean room
- What types of analytics each party can perform on the data
- What data, if any, can leave
Here is a tl;dr list of data clean room use cases:
- Is publisher xyz a good place for me to advertise?
- Is Connected TV network xyz a good place for me to advertise?
- Attribution: did our partnership with company xyz produce conversions?
- Build up contextual data about your users
- Media planning: analyze the intersection between your viewership and
advertiser audiences - User retargeting
- Audience amplification
- Marketing activation
- Marketing analytics
Use case: is publisher XYZ a good place for my org to advertise?
Let’s say you’d like to target a user who has opted in to your CRM with the email janedoe@mail.com, and you want to know if you’ll likely find her on a major publisher site [it could be, for example, vice.com or nyt.com].
You and the publisher can both share your email lists with the data clean room platform, which will compare the lists and report back on percentage overlap between them. If there is, say, a 95% overlap between your list and the publisher’s, the chances of reaching the customer tied to janedoe@mail.com is high. You and your publisher partner can go through a similar process for analytics after the fact, comparing your target customer data with the publisher’s information about registered visitor behavior. (Mediawallah.)
A CTV use case example
The Trade Desk’s recent clean room data integration with Disney is a good example. Using the Disney clean room product – which is backed by Snowflake, Habu and InfoSum – advertisers that buy through The Trade Desk can target audiences in CTV households by matching to Disney’s identity graph. Advertisers can use Unified ID 2.0 IDs (programmatic web IDs based on emails and phone numbers) to target those audiences across Disney properties.
A marketing attribution use case
Acrisure, an insurance company, just bought the naming rights to the NFL stadium of the Pittsburgh Steelers, which has been called Heinz stadium for more than 20 years. If Acrisure wants to attribute sponsorship value, it might do a data clean room integration with Ticketmaster, say, or maybe even with CPG company Kraft-Heinz itself to see if Heinz Stadium fans got behind the change. After all, Kraft-Heinz and other consumer brands that previously never owned much first-party data of their own are now doing whatever they can to get it, specifically to take advantage of products like clean rooms.
An advertising planning use case
Let’s say a brand has its own first-party data containing attributes about its customers and their associated sales SKUs. The brand wants to advertise to find new customers with the same attributes, and to combine those attributes with other characteristics to drive upsell opportunities. To create the target segments and comply with privacy requirements, the brand uploads its data into a clean room operated either by the brand or its ad partner. Participants can securely join any first party data without exposing IDs.
In a scenario like this, only limited amounts of data can flow between the various companies due to data privacy regulations and competitive concerns. In particular, it has been difficult to link a campaign with a business outcome for a customer. Analyzing this data can be opaque and inefficient because parties cannot share personal information about individuals, and due to the strict controls between online and offline advertising.
To make analytics more efficient and real time, and to allow for deeper analysis, a company can configure a [data clean room] where the participants can privately “list” the data that would be useful for this analysis—in a place where only the right parties can see it—without moving the data out of its database account. Each participant then can configure access controls, use secure functions, and leverage secure joins to properly protect the data, while still permitting joint data analysis… an advertising platform could use secure functions to allow analysis of advertising displays by geography, but prevent results from geographic areas or sample sizes that are too small. Secure functions can also disallow queries that may violate privacy principles.
And secure joins can establish linkages at the individual level (people, devices, cookies, or other identifiers) without exchanging or making visible any PII.
Data clean rooms and Roqad
We partner with a couple of the most trusted and respected data clean room providers.
The Roqad identity graph can be applied on top of a data clean room to potentially increase the audience segment for the data clean room participants.
And/or we can set up the data clean rooms as a trusted partner.
Here is an example: let’s say a car company has 10 million IDs in their first-party data set, and a global news publication has 10 million of their own IDs.
The two companies can put their IDs in a data clean room and see how much overlap they have. Let’s say that they do so, and the overlap is 5 million IDs. Great! Now they can do targeting and analytics on the 5 million IDs. The Roqad identity graph can be applied and increase the 5 million segment by another 50%.
We can also take inputs in a clean room from our own logs and our customer’s logs, apply our algorithm inside the clean room, and deliver the graph without ever seeing any of our customer’s data at all.
All parties benefit.
We do a lot of this kind of thing – apply the identity graph and the segment is extended by X%, meaning growth and insights for our partners.
Send us a message, ask a question!
References
“Adexplainer: Data clean rooms.” By James Hercher.
The Data Deprecation Glossary Every Marketer Needs,” by Forrester staff: Tina Moffett, Stephanie Liu, Joe Stanhope, Fatemeh Khatibloo.
“Habu State of Data Collaboration Report 2022.” By Ascend2.
“Distributed Data Clean Rooms Powered by Snowflake,” by Justin Langseth.
“Disney Integrates With The Trade Desk And UID2 In Pursuit Of Better Addressability.” By James Hercher.
“MediaWallah Clean Room Buyer’s Guide and Workbook.” By MediaWallah staff.
“The Ultimate Guide to Data Clean Rooms.” By InfoSum staff.